Using Analytics to Derail
Fraud Before It Happens
Advanced cognitive solutions could potentially enable organizations to assess
fraud and corruption risk on a broader scale, with far greater precision and
efficiency, as discussed in a recent Deloitte webcast,
The Future of Investigations: Derailing Fraud Before It Happens.
The new approaches to risk management of fraud and corruption are being driven
by rapid changes in analytics. “This advanced technology — in the hands of
experienced investigators and paired with the abilities of data scientists and
other analytics professionals — enables the organization to take the fight
against fraud to a new level,” says
Don Fancher, a principal at Deloitte Financial Advisory Services LLP and
Deloitte Forensic’s global leader.*
There may well be a regulatory benefit as well, since U.S. regulators have
invested significantly in analytics to better identify potential fraud schemes,
and increasingly expect organizations to utilize advanced technology to monitor
potential fraud and corruption risks.
“Regulators will, of course, be interested in an organization’s response to
known issues, but also will likely inquire about technology tools and solutions
designed to understand the entity’s fraud and corruption exposures based on
industry, geography, and business practices. Building preventative and
predictive analytics solutions as part of an overall compliance system may serve
to reduce potential liability when wrongdoing is discovered,” according to
Ed
Rial, a principal at Deloitte Financial Advisory Services LLP and Deloitte’s
U.S. Investigations leader.*
The Importance of Listening
Identifying vulnerabilities to fraud and corruption through root cause analysis
— and taking corrective action by building a monitoring and sensing capability
into the organizational infrastructure — can serve to protect the organization
by preventing wrongful conduct before it takes hold. “Analytics now present the
ability to discern faint signals that may represent outlier conduct across large
and diverse data sets,” adds Rial.
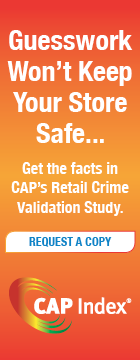
“While there is plenty of talk about big data solutions, not everybody
understands what that means: It requires the organizational openness to hearing
what the data is saying, rather than heeding preconceived notions about key risk
indicators,” notes Doug Veivia, vice president at Prudential Financial and a
member of Prudential’s international insurance compliance team. “To be ready to
accept what the data is indicating is critical. Sometimes the toughest challenge
is having the right people with the requisite business knowledge and openness to
approaching problems and being informed in different ways. And data analytics
helps address that challenge.”
Building an Analytics Program for Detection of Fraud and Corruption
When starting to build an analytics program for fraud detection, it’s critical
that the organization know what it is trying to solve for, settle on key
questions to address, and determine the scope: If the analytics program seeks to
solve every issue the organization faces, it could start running in circles with
no output.
“Building an analytics program requires strategic planning, a road map and a
combination of people, technology and well-thought-out goals,” says
Satish Lalchand, a principal at Deloitte Transactions and Business Analytics
LLP and Deloitte Forensic’s analytics leader.** “There is no single tool that is
a silver bullet with respect to the issue. Rather, is it is a solution built
around a strategy,” he adds.
Four basic principles govern the building of a new analytics program (see chart
below). But it is important to keep in mind that such programs are not just
about data scientists, technology, software, or infrastructure; but rather the
people who have the insight. “A lot of this work still involves intuition and
the experience of having worked in the industry for long time and gaining the
core forensic skills required to understand when a problem is rising,” says Rial.
“To get started, an organization might consider performing a self-assessment in
order to establish a baseline of where it stands with respect to the type of
analytics it’s using, if it’s using any at all,” suggests Lalchand.
In general, there are six broad analytics techniques that organizations can
consider when building an analytics program: Rules, anomaly detection, machine
learning, visual analytics and dashboards, text analytics, and network analysis.
An organization can
use analytics techniques and build models in many ways, and using a combination
rather than just one may be better. “For example, there are many rules-based
approaches to flagging certain behaviors that are designed to address a specific
compliance or regulatory risk within the financial services industry. But for
the most part, these approaches are inflexible; they flag only behaviors that
are relatively easy to circumvent, and they are limited by the human bias that
is deciding what the rule is,” notes Fancher.
As an example of the benefits of using more than one analytics technique, one
organization wanted a model that not only would identify customer complaints,
but also predict when one might occur. The model began with identifying
customers who had submitted complaints, and via an application of predictive
analytics and text analytics, the model was able to analyze various aspects of
the behavior of those employees who were the subject of the complaints. The
model also looked at data such as commissions, earnings reprimands, and
compliance issues, and, ultimately, could predict whether an employee might be
associated with a certain type of fraud complaint.
“From a financial services perspective, two main goals tend to drive an
organization’s selection of which types of analytics capabilities to use,” notes
Veivia. “The first is protecting its customers, and the second is driving sales
growth. Having greater sensing ability can allow the organization to identify
troubling behaviors earlier — before there is real customer impact and then
regulatory — to inform how risk-monitoring rules should change going forward,”
he adds, noting that financial misconduct or criminal activity tends to evolve
over time.
“As we place procedures and controls in place, the conduct will evolve to
circumvent those controls. So what’s best for risk management is having an
ability to detect changing behavior that can then be identified or sensed
earlier, and addressed,” says Veivia.
Arriving at mature analytics is a measured journey. “Once an organization
understands the end goal, it can build a strategy and a roadmap for the
different capabilities it seeks,” suggests Lalchand. “The organization could
then take concrete steps, such as conducting pilots, working with smaller chunks
of data, or experimenting with models before making any larger decisions around
tools and approach,” he adds.
It’s important to note that when an organization does obtain findings through
analytics techniques, these findings merely represent leads. Further
confirmation is needed to identify that fraud is actually occurring. “But once
fraud is confirmed, the organization can feed the cases it has researched back
into the model — and thereby make the model more agile and smarter,” says Rial.
*Ed Rial and Don Fancher are
Deloitte Risk and Financial Advisory principals in the Forensic practice of
Deloitte Financial Advisory Services LLP.
**Satish Lalchand is a
Deloitte Risk and Financial Advisory principal in the Analytics practice of
Deloitte Transactions and Business Analytics LLP.
This article was originally published on
wsj.com |