NYC's
Compstat & Predictive Policing - Transformed American Policing
The Story - The Impact - How Prevention Became the Driving Force
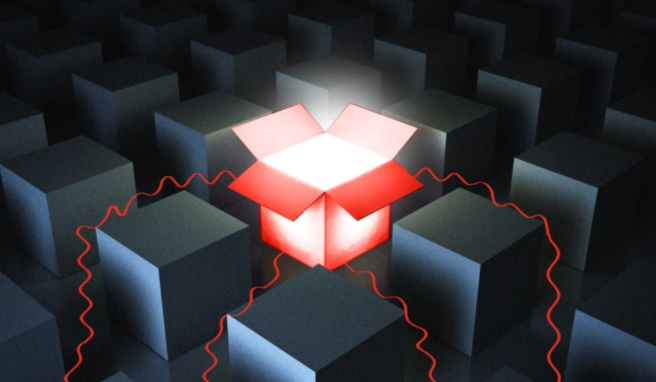
The black box of justice: How secret algorithms have changed policing
Crime prevention efforts increasingly depend on data
analysis about neighborhoods. But there’s a lot we don’t know—and vast
opportunities for bias.
The story of predictive policing begins in the 1990s with a process developed by
the New York Police Department. Today New York is one of the safest big cities
in America. In 2018, 289 people were murdered in the five boroughs. The city’s
murder rate—3.31 per 100,000 people—was the lowest measured in 50 years
In 1990, it was a different city: 2,245 people were murdered, a rate of around
31 per 100,000 (the city’s population increased markedly in the intervening 28
years). Here’s what the New York Times said about its hometown at the end of
1990: “The streets already resemble a New Calcutta, bristling with beggars. . .
. Crime, the fear of it as much as the fact, adds overtones of a New Beirut. . .
. And now the tide of wealth and taxes that helped the city make these streets
bearable has ebbed. . . . Safe streets are fundamental; going out on them is the
simplest expression of the social contract; a city that cannot maintain its side
of that contract will choke.” To stop the choking, the city knew it had to get
crime under control, but the police didn’t have the right information.
In 1993, New York elected its first Republican mayor in almost 30 years—an
ambitious former federal prosecutor named Rudy Giuliani. It may seem hard to
believe now, but back then Rudy seemed to have at least a modicum of political
nous. He ran a law-and-order campaign, and soon after taking office appointed
Bill Bratton, formerly Boston’s police commissioner, then head of New York
City’s Transit Police, to head the NYPD.
Bratton soon ran into a problem: he found that his new department had no focus
on preventing crime. At the time, that was not unusual. Police did not have
crystal balls. They saw their job as responding to crimes, and to do that, the
crimes had to have happened already. They were judged by how quickly they
responded, how many arrests they made, and how many crimes they solved.
Police did not have access to real-time crime data. And, as Lou Anemone, then
the NYPD’s highest-ranking uniformed officer, explained in a 2013 report, “The
dispatchers at headquarters, who were the lowest-ranking people in the
department, controlled field operations, so we were just running around
answering 911 calls. There was no free time for officers to focus on crime
prevention.”
So the department began using computers to crunch statistics. For the first
time, crime data became available in near-real time. The department also began
calling regular meetings, where commanding officers grilled captains and
lieutenants, asking what they were doing to combat crime in their precincts. The
department named this practice—the agglomeration and mapping of real-time crime
data, as well as the legendarily terrifying meetings—Compstat. Its progenitor,
Jack Maple, said it stood for “computer statistics or comparative statistics—no
one can really be sure which.”
Maple’s invention rested on four main principles: accurate and timely
intelligence, rapid deployment, effective tactics, and relentless follow-up and
assessment. It sounds simple, even obvious: of course police should try to
prevent as well as respond to crime; and of course, to do this effectively, they
will need as much data as possible. Neither of those ideas were obvious at the
time.
&uuid=(email))
At around the time that Compstat was put in place, crime began falling. I do not
intend to analyze, litigate, or even hypothesize about the precise causal
relationships of Compstat to falling crime. With apologies to Dorothy Parker,
eternity isn’t two people and a ham; it’s two criminologists arguing over the
causes of the late twentieth-century crime drop. Perhaps the key was altered
police practices. Perhaps it was changing demographics. Perhaps it was
regulations that got the lead out of household paints and gasoline. Perhaps it
was some combination of environmental, political, and demographic factors.
Determining the correct answer is, mercifully, beyond the scope of both this
book and my time on this planet.
Still, the fact is that Compstat transformed American policing (in much the same
way as, and not long before, data-driven approaches transformed baseball). Other
departments adopted it. New York has maintained and tweaked it. Today, a
major-metro police department that considers only response and not prevention,
and that purports to fight crime without data and accountability, is all but
unthinkable. Predictive algorithms seem to be the natural outgrowth of the
Compstat-driven approach: perfectly suited to departments concerned about
preventing crime, not just responding to it.
How such algorithms make their predictions is not clear. Some firms say that
publicly revealing the precise factors and weights that determine their
predictions will let criminals game the system, but that hardly passes the smell
test: a guy isn’t going to decide to snatch wallets on Thirty-Fourth Street
today because he knows his local police department uses XYZ Safety Program, and
their algorithm currently forecasts high crime—and hence recommends increased
police presence—on Thirty-Eighth Street.
The algorithms are proprietary, and keeping them secret is a matter of
commercial advantage. There is nothing inherently wrong with that—Coca-Cola
keeps its formula secret, too. And, as I said earlier, there is nothing
inherently wrong with using algorithms. But, as Phillip Atiba Goff of the New
York University’s Center for Policing Equity said to me, “Algorithms only do
what we tell them to do.” So what are we telling them to do?
Jeff Brantingham, an anthropologist at the University of California, Los
Angeles, who cofounded PredPol, told me he wanted to understand “crime patterns,
hot spots, and how they’re going to change on a shift-by-shift or even
moment-to-moment basis.” The common understanding of the geography of street
crime—that it happens more often in this neighborhood than that one—may have
some truth in the long run, but has limited utility for police shift commanders,
who need to decide where to tell their patrol officers to spend the next eight
hours. Neighborhoods are big places; telling police to just go to one is not
helpful.
So PredPol focuses on smaller areas—those 150-by-150-meter blocks of territory.
And to determine its predictions, it uses three data points: crime type, crime
location, and crime date and time. They use, as Brantingham told me, “no arrest
data, no information about suspects or victims, or even what does the street
look like, or neighborhood demographics. . . . Just a focus on where and when
crime is likely to occur. . . . We are effectively assigning probabilities to
locations on the landscape over a period of time.” PredPol does not predict all
crimes; instead, it forecasts only “Part 1 Crimes”: murder, aggravated assault,
burglary, robbery, theft, and car theft.
PredPol is not the only predictive-policing program. Others use “risk-terrain
modeling,” which includes information on geographical and environmental features
linked to increased risks of crime—ATMs in areas with poor lighting, for
instance, or clusters of liquor stores and gas stations near high concentrations
of vacant properties. Other models include time of day and weather patterns
(murders happen less frequently in cold weather).
All of these programs have to be “trained” on historical police data before they
can forecast future crimes. For instance, using the examples above, programs
treat poorly lit ATMs as a risk factor for future crimes because so many past
crimes have occurred near them. But the type of historical data used to train
them matters immensely.
THE BIAS OF PRESENCE
Training algorithms on public-nuisance crimes—such as vagrancy, loitering, or
public intoxication—increases the risk of racial bias. Why? Because these crimes
generally depend on police presence. People call the police when their homes are
broken into; they rarely call the police when they see someone drinking from an
open container of alcohol, or standing on a street corner. Those crimes often
depend on a police officer being present to observe them, and then deciding to
enforce the relevant laws. Police presence tends to be heaviest in poor, heavily
minority communities. (Jill Leovy’s masterful book Ghettoside: A True Story of
Murder in America is especially perceptive on the simultaneous over- and
underpolicing of poor, nonwhite neighborhoods: citizens often feel that police
crack down too heavily on nuisance crimes, but care too little about major
crimes.)
Predictive-policing models that want to avoid introducing racial bias will also
not train their algorithms on drug crimes, for similar reasons. In 2016, more
than three-fourths of drug-related arrests were for simple possession—a crime
heavily dependent on police interaction. According to the Drug Policy Alliance,
a coalition that advocates for sensible drug laws, prosecutors are twice as
likely to seek a mandatory-minimum sentence for a black defendant as for a white
one charged with the same crime. I could go on for a few hundred more pages, but
you get the idea: America enforces its drug laws in a racist manner, and an
algorithm trained on racism will perpetuate it.
&uuid=(email))
Police forces use algorithms for things other than patrol allocation, too.
Chicago’s police department used one to create a Strate- gic Subject List (SSL),
also called a Heat List, consisting of people deemed likely to be involved in a
shooting incident, either as victim or perpetrator. This differs from the
predictive-policing programs discussed above in one crucial way: it focuses on
individuals rather than geography
Much about the list is shrouded in secrecy. The precise algorithm is not
publicly available, and it was repeatedly tweaked after it was first introduced
in a pilot program in 2013. In 2017, after losing a long legal fight with the
Chicago Sun-Times, the police department released a trove of arrest data and one
version of the list online. It used eight attributes to score people with
criminal records from 0 (low risk) to 500 (extremely high risk). Scores were
recalculated regularly—at one point (and perhaps still) daily.
Those attributes included the number of times being shot or be- ing the victim
of battery or aggravated assault; the number of times arrested on gun charges
for violent offenses, narcotics, or gang affiliation; age when most recently
arrested; and “trend in recent criminal activity.” The algorithm does not use
individuals’ race or sex. It also does not use geography (i.e., the suspect’s
address), which in America often acts as a proxy for race.
Both Jeff Asher, a crime-data analyst writing in the New York Times, and Upturn,
a research and advocacy group, tried to reverse-engineer the algorithm and
emerged with similar results. They determined that age was a crucial determinant
of a person’s SSL score, which is unsurprising—multiple studies have shown that
people tend to age out of violent crime.
Shortly before these studies were published, a spokesman for the Chicago Police
Department said, “Individuals really only come on our radar with scores of 250
and above.” But, according to Upturn, as of August 1, 2016, there were 280,000
people on the list with scores over 250—far more than a police department with
13,500 officers can reasonably keep on its radar. More alarmingly, Upturn found
that over 127,524 people on the list had never been shot or arrested. How they
wound up on the list is unclear.
Police have said the list is simply a tool, and that it doesn’t drive
enforcement decisions, but police have regularly touted the arrests of people on
the SSL. The algorithm’s opacity makes it unclear how someone gets on the SSL;
more worryingly, it is also unclear how or whether someone ever gets off the
list. And the SSL uses arrests, not convictions, which means some people may
find themselves on the list for crimes they did not commit.
An analysis by reporters Yana Kunichoff and Patrick Sier published in Chicago
magazine found that just 3.5 percent of the people on the SSL in the dataset
released by the CPD (which covered four years of arrests, from July 31, 2012, to
August 1, 2016) had previously been involved in a shooting, either as victim or
perpetrator. The factors most commonly shared by those on the list were gang
affiliation and a narcotics arrest sometime in the previous four years.
Advocates say it is far too easy for police to put someone into a
gang-affiliation database, and that getting into that database, which is 95
percent black or Latino, reflects policing patterns—their heavy presence in the
mostly black and Latino South and West Sides of the city—more than the danger
posed by those who end up on it. The above analysis also found that most black
men in Chicago between the ages of twenty and twenty-nine had an SSL score,
compared with just 23 percent of Hispanic men and 6 percent of white men.
Perhaps mindful of these sorts of criticisms, the city quietly moth- balled the
SSL in late 2019—and, according to the Chicago Tribune, finally stopped using it
in early 2020.
Article originally published on
fastcompany.com
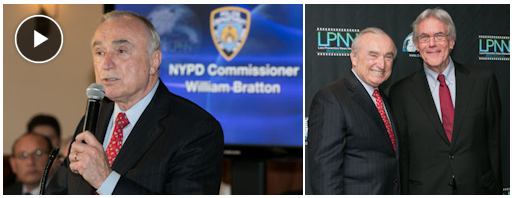
Commissioner Bratton spoke to the LP community at the
Daily's
'Live in NYC at the NRF Big Show' event in 2016.